Simple is good: How Francis Bach seeks to make machine learning research more accessible

At the recent NCCR Automation Symposium, we had the honour of speaking with Dr Francis Bach, a researcher at Inria (France’s National Institute for Research in Digital Science & Technology). Not only has Bach won a raft of prestigious awards, but as co-editor-in-chief of the Journal of Machine Learning Research (which runs on a groundbreaking fully free publishing model), and sometimes chair of the International Conference in Machine Learning, he is one of the leading lights of the machine learning research community. And taking an overview of Bach’s work on all these fronts, it becomes clear that accessibility is a passionate concern.
For example? Bach maintains a blog, where he takes a lighter, even playful approach to technical topics. “It’s like giving a talk,” he explains. “What I put in the blog is exactly what I say in my talks. It’s formal, but not too formal. You’re allowed to wave your hands to explain something, and draw pictures.” The formality of published papers is something of a bugbear for Bach. “It tends to be overly formal, at the expense of understanding. The goal of the blog is to say whatever I want, regardless of what is expected from a paper.”
That’s not about laziness, though. The lack of formality is how he connects with his audience. “In very cynical terms, I achieve much stronger communication with my blog than with going to a conference. In most of my blog posts, there’s a tutorial part, and then I squeeze in more of my work. I talk about things I like, so I end up talking about what I do. This is really a very efficient way to present topics that you like. It’s more efficient than giving a talk.”
Of course, a blog post is not a replacement for other modes of disseminating research – but it’s a valuable complement to other channels. “I consider a blog post for, let’s say, small, cute ideas. I don’t want to write absolutely the best paper, but I want the idea to be out. So I put it in a small blog post. Typically, I would write an arXiv paper with all the formal maths, which is completely correct, and the blog post, but I may not publish it anywhere else.”
Bach is aware that this is something of a well-earned privilege. “I’ve reached an age where having an extra paper in a journal doesn’t make a big difference for me.” He has similar feelings about conferences. “When I started in machine learning, the main conference was like 200 people. Now it’s more like 10,000 or so. It’s very big and overwhelming, and I think the interactions are not as strong as before. Since I’m old enough to have already acquired a network of collaborators and friends, I don’t need it.”
Bach is also convinced of the environmental importance of reducing air travel, although sometimes it can’t be avoided. “I still had to go to Japan this year, for the big applied maths conference (ICIAM). Some invitations we cannot refuse.” And neither can those less advanced in their careers. “As a young person you have to go to a few meetings – I don’t believe in research in isolation. As a young researcher, the only way you get to meet people is to go to seminars or symposia, like this one, or conferences. But for me, I don’t need it so much any more. I like to see my friends, but I can see my friends in smaller venues in Europe.”
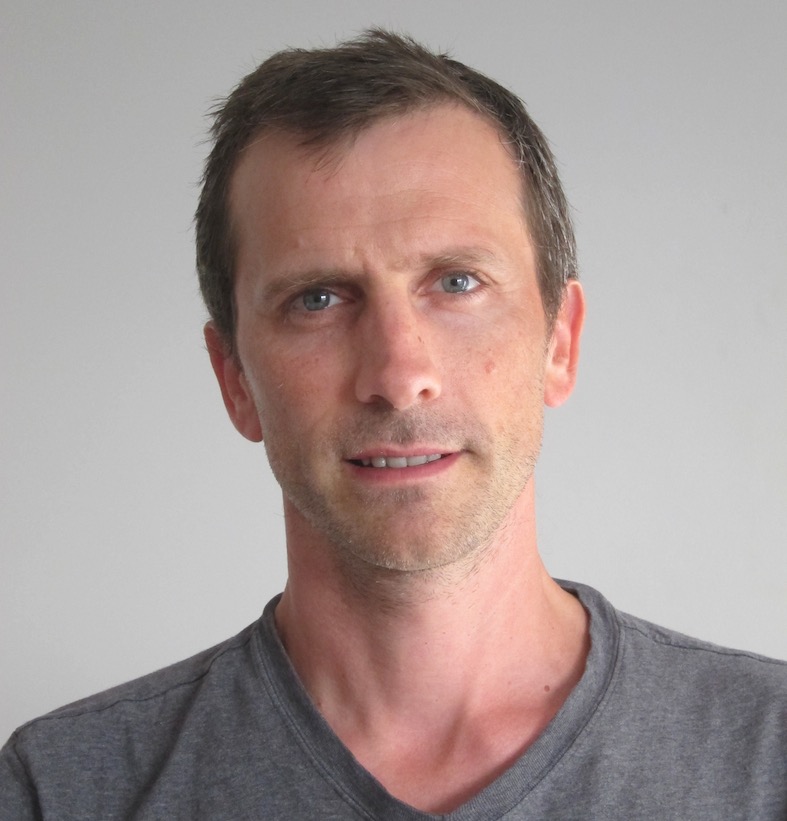
The pressure of a popular field
This awareness of the demands on younger researchers echoes Yurii Nesterov’s complaints in our recent interview about the pace of academia today. Bach agrees with that. “You need to publish faster, much faster than when I was a student. So you have many people working on the exact same topic. I try to avoid those crowded fields. If I know that 20 people are doing the exact same thing, why bother? Let’s try to focus on things which are not as mainstream, but interesting.”
Again, this may be harder for younger researchers. “Students need to have a job, so you need to publish. But if you are a student working on a topic, and you see the arXiv papers coming out every week or every day, and you see your research topic, it’s very stressful. This happened to me once, that I got scooped. I was angry; that’s fine, that’s life, and probably I’ve done it to other people accidentally. It’s a good thing to be in the open, to be read. You need to be exposed to other people doing the same thing. But try to avoid the topics that everyone is working on.”
ArXiv has emerged to fill the gap between submitting a paper for publication and actually seeing it released (perhaps some years later). But this comes with pros and cons, even beyond the accelerated pace. “You have your idea, you put it in arXiv, so that’s good because it’s disseminating ideas. But this is before peer review; this is an issue, this is never clear. So the paper is out there, people can make up their own minds. When I read a paper on machine learning, I don’t need reviewers to tell me if it’s good or not. But if you’re a bit of an outsider, or maybe a student, you should not take arXiv submissions as being peer reviewed.”
It’s still progress, though. “I think it’s for the better. I prefer arXiv submission and then peer review to delaying publication a few years. Personally, it’s very hard for me not to talk about my new paper. And if there’s an embargo – to me that’s insane. Even Nature is giving up on embargo because of arXiv.”
The fight for academic freedoms
This ties to the scorching hot topic of open access publishing. Nature and other bastions of science have come under fire for clinging to the traditional model of academic publishing, keeping papers behind a paywall that tightly restricts the spread of knowledge. The Journal of Machine Learning Research (co-edited by Bach) is different.
“On other journals, you can pay to make your paper open access. You have to pay $4,000, or sometimes five or six or ten. The model of JMLR from the start was totally free. No cost of any kind. It’s open and available for everybody.”
Extending this model to other journals would demand commitment from the editors – such as that shown by the founding editors of JMLR, who launched the journal in reaction to restrictive policies at another publication. “Half the board quit and created JMLR, which is now by all standards much better than the older one. This required leadership from the community, for members of the community to say this is enough, we can set up our own journals. It worked.”
Perhaps surprisingly, cost is not the big obstacle to a free publishing model. “We don’t have external copy editors. We don’t print. The human cost is the problem, so we have only volunteers. From editor-in-chief to editors to reviewers, everything is voluntary; it’s the same in most journals. The hard work is volunteer work – some money is made but not given back to the actual people that do the job. And there are some admin costs, which we pay from grants and gifts.”
What makes it work is commitment from the community – and the fact that it is still a rather new field. “It makes it easier,” says Bach. “We have no big society trying to control us. This impacts everything that we do.”
He enjoys another kind of freedom, too. In choosing topics, Bach is guided by his curiosity as well as by where he sees a need. “When you see a medical doctor who wants to do something with machine learning, but we don’t have the ability yet – this leads to developing new frameworks for machine learning. That’s one area, interaction with others. But also, the other area is personal taste. It’s learning new things. Learning new toys to play with. This is the good thing about mathematics: the quantity of things you don’t know is far bigger than the things you know.”
Borrowing tools can drive breakthroughs
Pursuing this curiosity can also turn up unexpected connections. There are so many topics, “and they may look irrelevant, for your particular interests. But you discover that in fact, there are vast amounts of literature, covering exactly what you want to do, with different names.”
So what was the last finding that surprised him? He points to optimization, a field in which (as >we have explored on the NCCR Automation researcher blog), convexity is usually understood to be the ultimate guarantee of robust results. But it can be elusive. “You may have properties which can satisfy a solution for optimization, but not convex,” he says. “Neural networks, which are the key framework at the moment for AI and machine learning, lead to those types of problems, where things are hard to satisfy. You launch, you hope for the best, and it works, but not everybody understands why. And now people are finding ways of showing that you do get the solution in robust ways. I think that’s a great achievement – how you can finally, after exploring new types of mathematical tools, get your hands on guarantees for neural networks.”
Again, borrowing ideas from other research is key to progress. “This is a very nice example of a problem that seems very hard at first. If you stick with the tools you have, you’re crazy, it won’t work. But there are other tools that can be used. We leverage tools from partial differential equations and so on to get some sort of guarantee.”
More surprises are on the way, he expects. “You see more and more of that. People observe some behaviour on neural networks, and then finally find a way of explaining why it works. And once you know why it works, it gives you an idea how to improve them and make them more robust. This is where theory can have an impact.”
Does this solve the problem of black-box machine learning – the concern around relying on machines in high-stakes decision making, when we’re not really sure how the machine is making those decisions?
<“There are two aspects here. You may want to have some certification of what the neural network does. If you put it in cars and planes and hospitals, you need to certify what it does. Which is important. But what I'm more concerned about is, how can it learn from data? How can it achieve that kind of behaviour? Achieving the behaviour, and explaining and certifying the correctness of that behaviour, are two different things.”
He elaborates: “What data should you feed your neural network to achieve a given task? Do I need to label one million or one billion sentences to do machine translation? That is important, and then being able to certify that the machine correctly translates the sentence is a different question. Those two are a bit related. It’s very important to know how it does it, and it’s especially important to know what data it was trained on.”
In talking to Nesterov recently, of course, we heard that he had very strong opinions about non-convex optimization, saying that non-convexity simply showed “we didn’t think enough”. Bach, naturally, doesn’t quite agree. “I agree with the principle that if you don’t look at the problem in the correct way, you aren’t able to show that it works.”
But convexity isn’t the right approach for neural networks. “There are still local minima or things where you can get trapped. Convexity is really, wherever you start from, you go down and you’re going to end up where you want. But with neural networks, you have to start in a good location. You can show that you can avoid those traps, but it depends on where you start. This is one example where it’s not only about being convex – it’s a bit more than that. I agree that finding convexity is important, but this cannot be the only tool.”
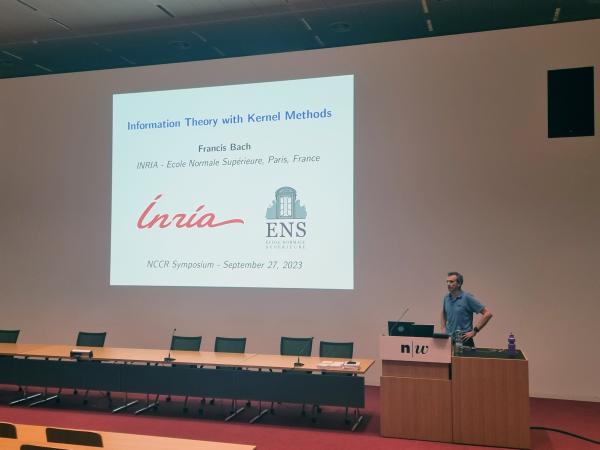
Simplicity is the goal
Bach’s big project right now is a book on machine learning theory for graduate students. The idea, he tells us, is to be as simple as possible, and explain everything from first principles. “The idea is to take students where they are, with a good level of mathematics but not too much, and allow them to answer simple questions. Why do learning techniques work? What doesn't work? How many data points do I need?”
The book will be written “in the simplest possible terms” and without too many formulae. “There are formulae of course, but the idea is not to be the state of the art of the latest work, but work that can be understood by people with a reasonable but limited mathematical background.”
Bach takes an obvious joy in making his topics accessible. Simplicity, he says, is a virtue. “When I say something is simple, it's good. But, I would say, some papers try to overcomplexify. This I don't like so much. I believe in simple ideas. If the idea is simple, it can be proved in a simple way.”
Interview and text by Robynn Weldon.