Mahrokh Ghoddousi Boroujeni
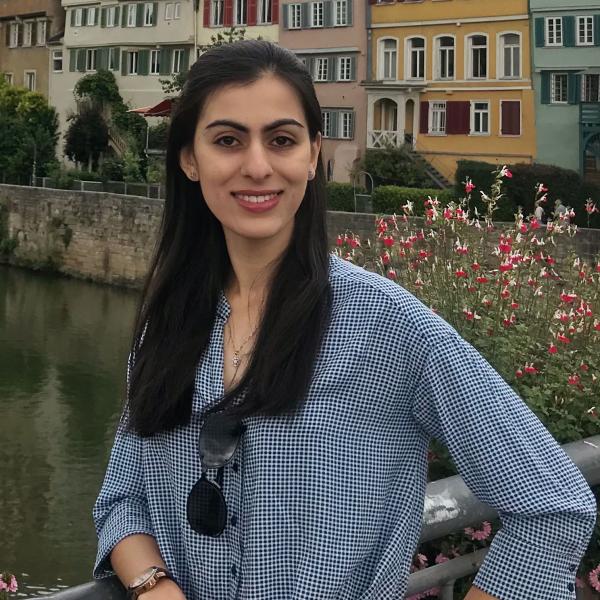
Mahrokh Ghoddousi Boroujeni
PhD Student
I want to leave Earth a better place by contributing to science and the environment, and NCCR Automation is the way for me to do both.
Mahrokh Ghoddousi is a doctoral assistant at DECODE, EPFL, jointly supervised by Prof. Giancarlo Ferrari Trecate from EPFL and Prof. Andreas Krause from ETHZ. Mahrokh holds two BSc degrees in Electrical Engineering and Computer Science from Sharif University (Iran). She previously did research internships at IFA (ETHZ), PVLab (EPFL), and Movement Generation Lab (Max Planck Institute).
Mahrokh has taken part in the #NCCRWomen campaign. You can see her great video here.