Muhammad Zakwan
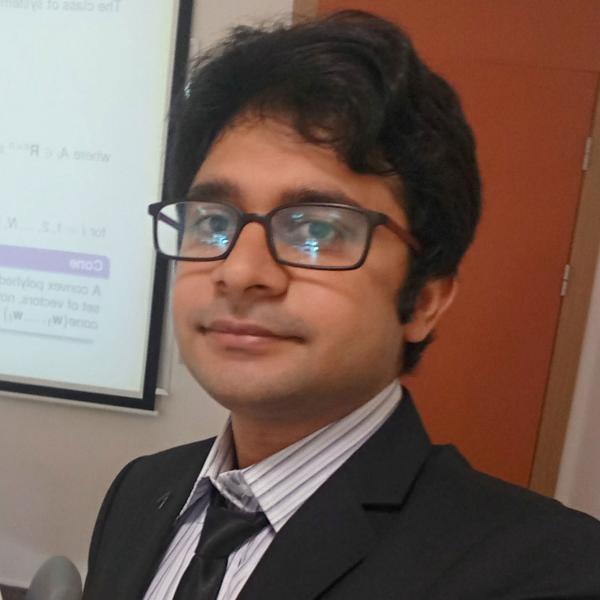
Dr.
Muhammad Zakwan
PostDoc
Realizing mathematical constructions to tangible real-world applications for a better future.
Muhammad Zakwan holds a dual position as Team Leader in AI and Control at Inspire AG in the Control and Automation group with Efe Balta, and Postdoc working Efe Balta, Alisa Rupenyan, and John Lygeros.
Prior to that, Muhammad Zakwan completed his PhD in the Dependable Control and Decision group (DECODE) at École Polytechnique Fédérale de Lausanne (EPFL) (also within NCCR Automation). Zakwan holds an Electrical and Electronics Engineering degree from Bilkent University (Turkey) and a Bachelor of Science in Electrical Engineering from the Pakistan Institute of Engineering and Applied Sciences (Pakistan). His research interests include LPV systems, hybrid dynamical systems, time-delay systems, distributed systems, and machine learning.