Philipp Heer
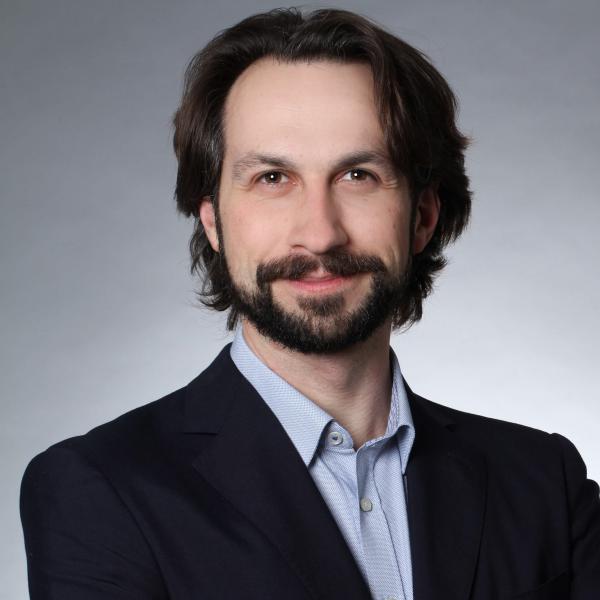
Philipp Heer
PI
It is truly exciting to develop solutions towards a sustainable energy system for society by the means of collaboration and applied research in control.
Philipp Heer is the deputy head of the Urban Energy Systems Lab (UESL) at Empa - an Institute of the ETH Domain and is responsible for the energy and digitalization related research at the Empa demonstrators NEST, move and ehub. He received a BSc from HSLU T&A (2010) and a MSc from ETH Zurich (2013) both in Electrical, Electronics and Communications Engineering and a MAS from ETH Zurich (2018) in Management, Technology, and Economics. His research focusses on developing operational solutions based on data-driven approaches to foster a sustainable energy system.