Matteo Turchetta
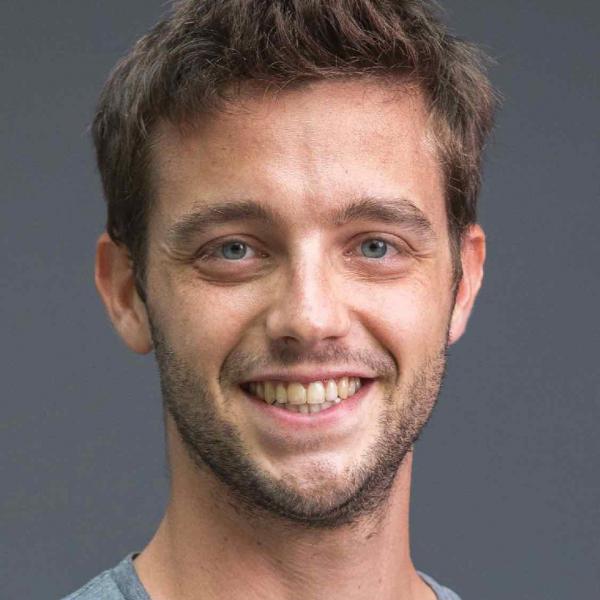
Matteo Turchetta
PostDoc
Alumni
I believe that safety and robustness of learning systems is one of the most exciting challenges we face to embed smarter and smarter systems in our daily life.
Matteo Turchetta is a postdoctoral researcher at the Learning and Adaptive Systems group at ETH Zurich. His research focus lies at the interplay between control theory and machine learning. In particular, he aims at building provably safe learning agents capable of making decisions in safety-critical dynamical environments under uncertainty.
After completing his Postdoc, Matteo has gone on to build his start-up, Kora Labs.