Alisa Rupenyan
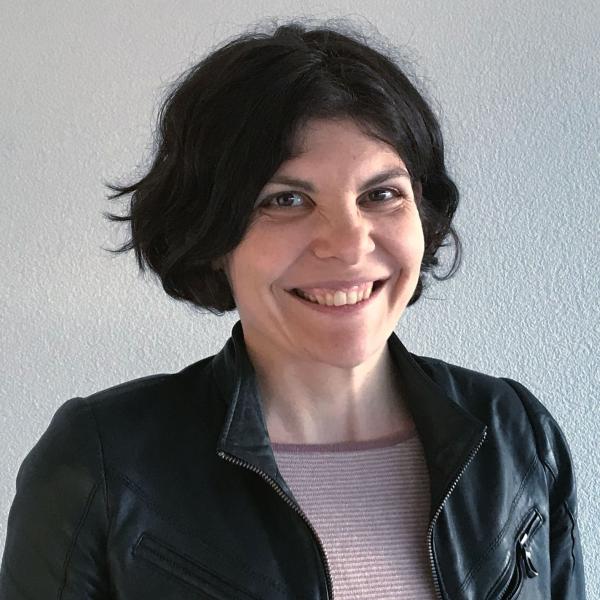
Dr.
Alisa Rupenyan
PI
I’m excited about interdisciplinary research connecting machine learning and control theory, and opportunities for impact in major application domains.
Alisa Rupenyan is the Rieter endowed professor at the Zurich University for Applied Sciences (ZHAW) and Head of the Industrial AI group at the ZHAW Center for AI. She leads research projects in the intersection between process optimisation, industrial control, and machine learning for applications strongly connected to manufacturing.
Previously, she led application development in the Zurich-based startup Qualysense AG for fast grain-sorting robots using machine learning techniques for high-dimensional data. Her Ph.D. is in the field of time-resolved laser spectroscopy from the Vrije Universteit Amsterdam (2009) and her MSc degree is in Laser Physics from the University of Sofia.