Seven new industry collaboration projects funded

OPFV2: Automated incentives for voltage support procurement
Project lead: Saverio Bolognani
A new challenge for the Swiss transmission system operator Swissgrid is to regulate the grid voltage in the presence of an increasing number of small-sized distributed generators replacing large traditional power plants. The innovative solution deployed by Swissgrid consists of procuring voltage support services from the distribution grids: distribution grid operators are provided with voltage references and are asked to tap into their local reactive power resources to track that voltage. In exchange for this service, they are financially remunerated. Unfortunately, a preliminary study that we conducted based on Swissgrid data shows that the resulting quality of the voltage support service is lower than expected. We identified two main causes of this suboptimal performance. First, a proper formulation of the control problem should include a model of the reward-seeking behavior of the distribution grid operators. In other words, the financial reward should not be designed ex-post as compensation for participation but has to be considered as part of the control signal and studied as such. Second, the current decision architecture lacks feedback. Even if the response of the distribution grid can be observed, it is not used to improve the next control signal and, most importantly, it is not used to learn the state of the flexible resources that reside in the distribution grids and that Swissgrid is trying to tap on.
The project proposes upgrading the decision algorithm that currently determines the voltage set points for all distribution grids so that it incorporates adaptive functionality that allows it to learn the grid's state and adjust the decision accordingly.
The co-design of this automation/incentive scheme requires tools that typically fall under "intervention in games" and "feedback optimization." These methods must be validated in simulations to be engineered into a real-time algorithm that can be incorporated into Swissgrid operational decisions. This, in turn, requires the development of data-based models for the rational response of distribution grid operators. If the proposed solution outperforms the current one in simulations, it can be tested on streams of real online data and then deployed in the real grid. The project's goal is to permanently incorporate the proposed learning/adaptation algorithm into Swissgrid operations.
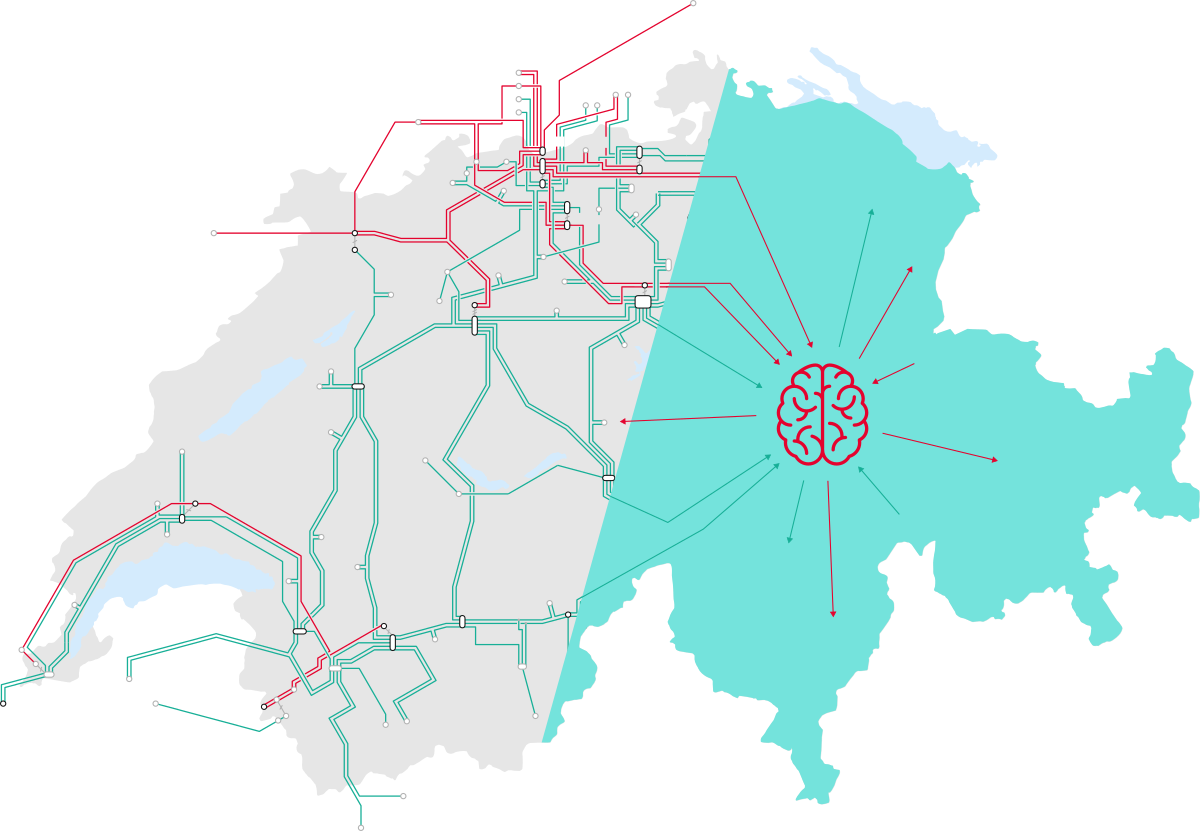
COORD: On-demand fleet orchestration and coordination with existing transit network operations
Project lead: Nikolas Geroliminis
In 2022, Public Transport of Fribourg (TPF) transported 34.5 million passengers across its 66 bus routes and 3 rail lines, with this number continuously on the rise. TPF is expected to face important challenges in the years to come due to the growth of demand, efforts towards environmental impact and sustainability and integration of new technologies in efficient, sustainable, and reliable operations of public transport. Similar trends and challenges apply to almost every urban area in CH and worldwide. This project intends to revolutionize how emerging technologies and their applications reshape public transport services by providing flexible collective transport with increased accessibility, sustainability and reliability. COORD aims to integrate in real-time multi-sensor public transport data with Machine Learning, traffic modeling and optimization to comprehensively explore the benefits of flexible on-demand transport for public transport improvement. Emerging transportation paradigms like dedicated and dynamic bus lanes and on-demand services, coupled with strong forecasting and optimization methods will create more reliable, efficient and sustainable public transport services. TRANSFORM will pioneer a unique blend of quantitative methods and engineering principles to create a one-of-a-kind multi-sensor mobility observatory for high-quality monitoring, prediction, and management of public transportation in the city of Fribourg with a strong interest from the city for a field implementation. The project will develop two parallel themes. Thread 1 focuses on the optimization of station-based on-demand micro-transit services as a first- or last-mile solution for public transit. Thread 2 aims at conceptualizing on-demand services as an interconnected transport alternative to fixed-line buses in instances of demand surge or congestion uncertainty. The synchronization and coordination of fixed- and flexible-line services will result in improved services even in case of abruptions and non-recurrent events. The project aims to employ novel and often disruptive technologies to solve pressing mobility issues (congestion, livability, sustainability, safety and equity) for the city of Fribourg. The developed framework will be generic, scalable and adaptable for public transport management and services to enhance mobility at urban and interurban levels to other Swiss cities and worldwide. For transport authorities and municipalities, COORD provides understanding, methods, models and algorithms for multi-modal traffic management.

Optimized Coordination of Portfolio of Co-Located Renewable and Storage Resources
Project lead: Gabriela Hug
The electric power system is currently in a transition from a supply system composed of a few large flexible power plants to many variable distributed generation resources based on wind and solar energy. Hence, the system is rapidly changing, and the volatility of the new resources bring challenges to grid operation in terms of balancing but also in terms of grid congestion. Storage devices, such as batteries, facilitate the penetration and support the integration of variable renewable generation. Co-locating batteries with renewable energy sources has multiple advantages such as local coordination potential between generation and storage resources, saving on interconnection costs and possibly even more important reducing the permitting times. However, the existing grid interconnection capacity is limited and has been designed for accommodating the peak generation of the renewable resource. Energy supply companies furthermore need to deal with the situation that they have a portfolio of generation resources distributed throughout the grid. Hence, in this project, together with Alpiq, we study the situation in which a supplier has multiple PV and/or wind power parks with which he bids into the market. We develop a stochastic optimization approach which provides as an outcome the optimal bids across multiple markets for a portfolio of renewable resources, some co-located with batteries, taking into account potential grid limitations. Furthermore, we close the loop and provide an approach to operate the distributed resources according to the cleared bids.
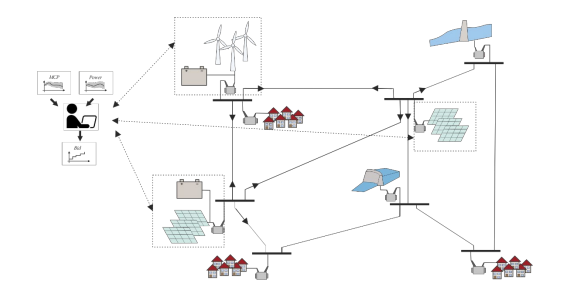
Advancing Supply Chain Efficiency and Sustainability through Data-Driven Innovation
Project leader: Daniel Kuhn, Andreas Krause, Yifan Hu
The retail industry is at a pivotal point where the integration of advanced analytics can significantly enhance operational efficiency and sustainability. On the other hand, many retail companies with specialty in perishable goods, currently utilize basic demand forecasting and load scheduling models. These models require manual interpretation by experienced managers, leading to potential inefficiencies, increased waste, and missed opportunities in a fast-paced market.
To address these challenges, we propose a comprehensive four-year research project. The project aims to transform the company's supply chain management through the development and implementation of advanced optimization, control, and statistical tools. The research focuses on three key areas:
1. Context-Based Demand Forecasting: We will develop sophisticated demand forecasting models that incorporate contextual factors such as seasons, holidays, and whether it's a weekday or weekend. By leveraging the company's extensive SQL-accessible database, these models will provide more accurate and nuanced demand predictions. This enhancement will enable the company to better align its inventory levels with actual consumer demand, reducing both stockouts and overstock situations.
2. Integrated Learning and Decision-Making for Inventory Control: We will create an integrated framework that combines real-time data analysis with sequential decision-making processes. By unifying estimation and optimization, the company can adjust inventory policies dynamically in response to the latest market trends and consumer behaviors. This integration reduces reliance on manual decision-making, increases responsiveness, and enhances
overall operational efficiency.
3. Enhancing Sustainability Through Matched Supply and Demand and Resale: With a significant portion of the company's products being perishable, minimizing waste is both an economic and environmental imperative. By optimizing supply chain management to better match supply with demand, we aim to significantly reduce product waste. Further considering the pricing and supply chain for the resale channel, this effort contributes to sustainability goals and aligns with growing consumer preferences for environmentally responsible businesses.
Implementing these advanced analytics tools will provide numerous advantages:
• Improved Accuracy: Enhanced forecasting models will reduce forecasting errors, leading to better inventory decisions to match the supply and demand.
• Operational Efficiency: Automated, integrated systems will streamline processes and free up managerial resources for strategic initiatives.
• Waste Reduction: Optimized inventory management will decrease perishable waste, reducing costs associated with unsold goods.
• Competitive Advantage: The use of advanced analytics will position the company as an industry leader, appealing to consumers who value reliability and sustainability.
Beyond the benefits, the project contributes to broader societal and environmental goals, including (1) environmental sustainability about reducing waste and minimizing the environmental impact associated with overproduction and disposal; (2) economic efficiency in potential lower prices for consumers and increased economic efficiency in the retail sector. The company would also enhance its reputation as a socially responsible company, meeting the growing consumer demand for ethical and environmentally friendly businesses.

High-dimensional safe control- and process parameter tuning with Digital Twins
Project lead: Alisa Rupenyan
This project aims to develop and validate a toolbox for high-dimensional data-driven optimization using Digital Twin (DT) technology to enhance efficiency in product design, operational excellence, and sustainability. Our toolbox targets data-driven optimization problems where performance metrics and constraints are directly measurable. Initial validation will use a robot arm at ZHAW. Algorithm development and testing will take place at ZHAW, with DT development on Siemens' Amesim platform. The algorithm will be further validated at the Siemens Digital Industries lab in Leuven, Belgium. For this, an autonomous vehicle will be used, where tuning the control parameters poses a significant challenge due to the controller’s complexity.
Our research supports NCCR Automation's objectives to advance automation tools that boost efficiency and sustainability. Phase I NCCR Automation research indicated DTs' crucial role in advanced manufacturing, particularly in energy savings.
This collaboration extends DT-based optimization methods for practical applications with significant impact on various manufacturing processes and stages. This collaboration aims to develop an advanced optimization toolbox using DT technology, benefiting various industries and SMEs through Siemens' extensive customer base and support infrastructure.

This project will explore data-driven tuning of high-dimensional controllers, production processes, and manufacturing equipment, where the data is collected with the help of Digital Twins of the systems of interest. It will incorporate also mechanisms for detecting when the systems start deviating from the Digital Twins, and adjusting the optimization to incorporate more data from the real systems.
Kuafu: An AI-based platform to unlock the energy flexibility of e-mobility and smart buildings for the energy transition
Project lead: Hanmin Cai
Grid operators are increasingly challenged by the intermittent nature of renewable energy sources, leading to significant costs and complexities in maintaining grid stability. To balance supply and demand effectively, they seek new ways to harness flexibility from local resources like electric vehicles and heat pumps. However, a communication gap with energy management system providers—who control these local assets—limits the potential to fully optimize grid performance. Kuafu offers an automated flexibility management platform as a customized intermediary between grid operators and energy management system providers. This platform facilitates efficient communication and coordination, enabling grid operators to leverage local resources better. Energy management providers gain valuable insights and opportunities to participate in grid services, enhancing their offerings to end-users through advanced automation systems. By bridging this gap, Kuafu's solution helps reduce operational costs and delays the need for costly grid reinforcements. It supports the integration of renewable energy sources, contributing to a more resilient and sustainable energy ecosystem. End-users benefit from optimized energy usage and the satisfaction of participating in a greener grid. We invite you to discover how Kuafu's innovative platform can transform flexibility management and deliver mutual benefits across the energy sector.

nuorail: numerical optimization solutions for energy-efficient trains
Project lead: Dimitris Kouzoupis, Francesco Corman
Railway transportation is inherently energy-efficient thanks to the low friction between steel wheels and track, which minimizes energy losses. Despite this advantage, the Swiss railway network - one of the most densely utilized in the world - consumes over 2 terawatt-hours (TWh) of energy annually, according to SBB. Given the scale of this consumption, even a small percentage reduction in energy usage can have a substantial social, environmental, and economic impact.
Currently, the railway industry predominantly relies on driver advisory systems (DAS) to ensure punctuality and energy efficiency. However, these systems are inherently constrained by the variability in driver performance. Meanwhile, although major train manufacturers are investing in the development of automatic train operation (ATO) solutions, most still rely on heuristic algorithms, and energy efficiency becomes more the focus of a marketing campaign rather than a genuine operational priority.
We aim to address these shortcomings by introducing state-of-the-art numerical optimization algorithms into the realm of railway industry and comparing the energy savings of our solutions against those of existing systems in a transparent and unbiased manner. Our journey began with the first NCCR Automation industry call, where we set out to bring our predictive control algorithms from scientific research to real-world application, in collaboration with Stadler and SOB. Since then, we have made significant strides. Our optimization software has been fully integrated into Stadler’s ATO system and rigorously tested through hardware-inthe-loop simulations. These simulations allow us to validate the performance of our algorithms in near-real-world conditions, ensuring that the system performs as expected under a variety of operational scenarios. Looking ahead, we are eagerly preparing for the first night tests, scheduled for January 2025. These trials, conducted on Swiss-made trains running on the Swiss railway network, will be a critical milestone in proving the effectiveness of our optimization algorithms in a real operational environment. The results from these tests will lay the groundwork for transitioning our pilot project into a commercially viable product.
Our vision extends beyond the initial implementation. We are committed to refining and evolving our solution based on feedback from train operators, manufacturers, and other key stakeholders. By engaging in collaborative pilot projects, gathering detailed feedback, and participating in workshops, we aim to fine-tune the product to meet the needs of the industry. Ultimately, our mission is to provide train operators with a robust, versatile tool that maximizes energy efficiency, reduces operational costs, and contributes to a more sustainable future for railway transportation.
