Daniel Kuhn
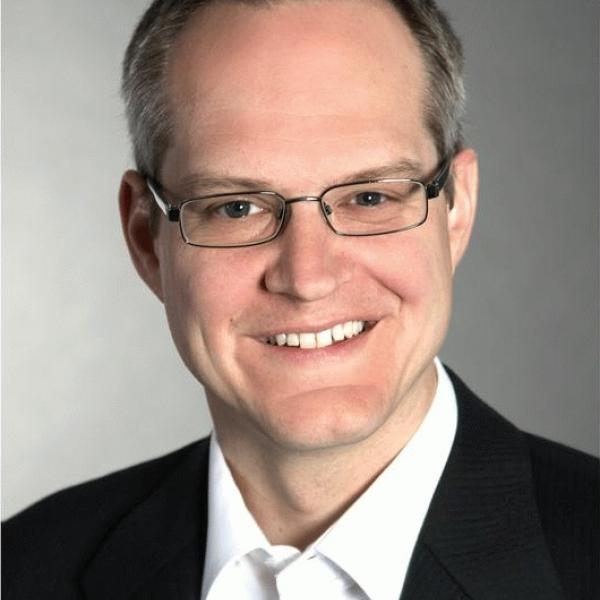
Prof. Dr.
Daniel Kuhn
PI
Aiming to optimize engineered systems affected by uncertainty, the NCCR Automation is right at the heart of my long-standing research interests.
Daniel Kuhn is Professor of Operations Research at the College of Management of Technology at EPFL, where he holds the Chair of Risk Analytics and Optimization. Before joining EPFL, he was a faculty member at Imperial College London (2007–2013) and a postdoctoral researcher at Stanford University (2005–2006). He received a Ph.D. in Economics from the University of St. Gallen in 2004 and an M.Sc. in Theoretical Physics from ETH Zürich in 1999. His research interests revolve around stochastic programming and robust optimization.