Tobias Sutter
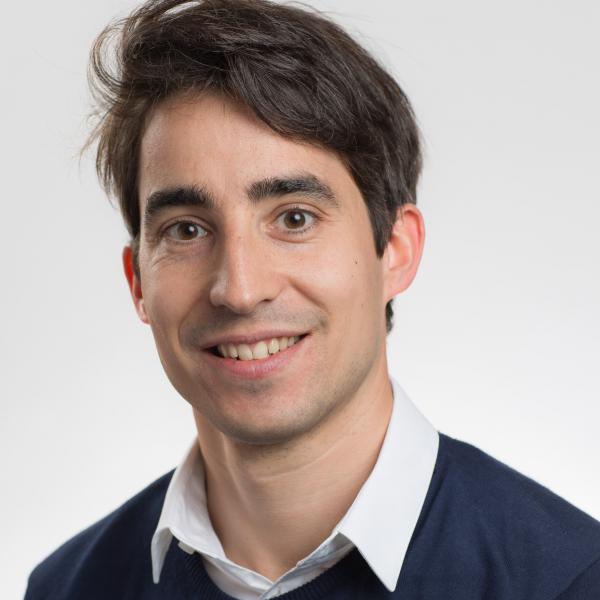
I am excited to work in the intersection of data science and automation with the goal to contribute towards a better theoretical understanding.
Tobias Sutter was a Postdoc at the Risk Analytics and Optimization Group at EPF Lausanne as well as the Learning & Adaptive Systems Group in the Institute for Machine Learning at ETH Zurich. He joined NCCR Automation from August 2020 to August 2021. He received his Ph.D. in Electrical Engineering from ETH Zurich (2017). He has been awarded the IEEE George S. Axelby Outstanding Paper Award (2016) and the ETH medal for outstanding doctoral thesis (2018). His research interests lie in the intersection of reinforcement learning, mathematical optimization and data science.
Tobias Sutter now works as assistant professor at the computer science department at the university of Konstanz.