John Lygeros
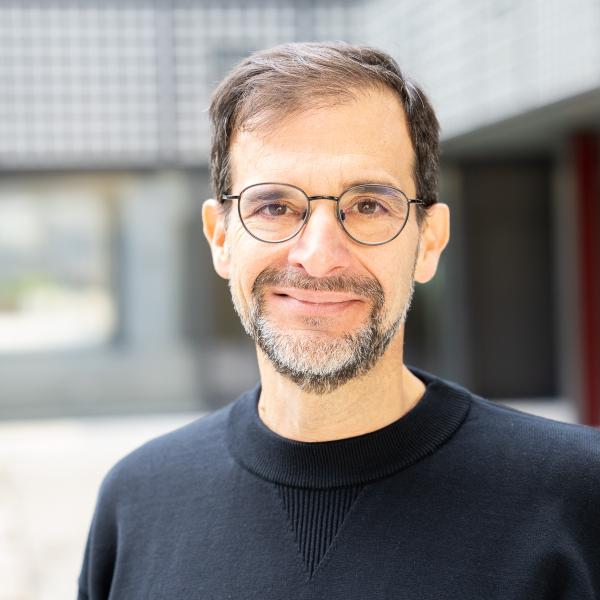
The NCCR changed my life and that of those around me.
John Lygeros grew up in Athens, Greece where he graduated from Athens College in 1987. He completed a B.Eng. degree in electrical engineering in 1990 and an M.Sc. degree in Systems Control in 1991, both at the Imperial College of Science Technology and Medicine, London, U.K.. In 1996 he obtained a Ph.D. degree from the Electrical Engineering and Computer Sciences Department, University of California, Berkeley. In the period 1996-2000 he held a series of research appointments at the National Automated Highway Systems Consortium, M.I.T., and U.C. Berkeley. In parallel, he also worked as a part-time research engineer at SRI International, Menlo Park, California, and as a Visiting Professor at the Department of Mathematics of the Université de Bretagne Occidentale, Brest, France. Between July 2000 and March 2003 he was a University Lecturer at the Department of Engineering, University of Cambridge, U.K., and a Fellow of Churchill College. Between March 2003 and July 2006 he was an Assistant Professor at the Department of Electrical and Computer Engineering, University of Patras, Greece. In July 2006 he joined the Automatic Control Laboratory at ETH Zurich where he is currently serving as the Professor for Computation and Control and the Head of the laboratory.
His research interests include modeling, analysis, and control of hierarchical hybrid systems, with applications to biochemical networks, large-scale systems such as power networks, surveillance systems and air traffic management.
John Lygeros is a Fellow of the IEEE, and a member of the IET and the Technical Chamber of Greece. He is currently serving in the IEEE Board of Governors, the Scientific Steering Committee of the Newton Institute, and as the treasurer of the International Federation of Automatic Control.