"Optimised control is central to a dependable automated future"
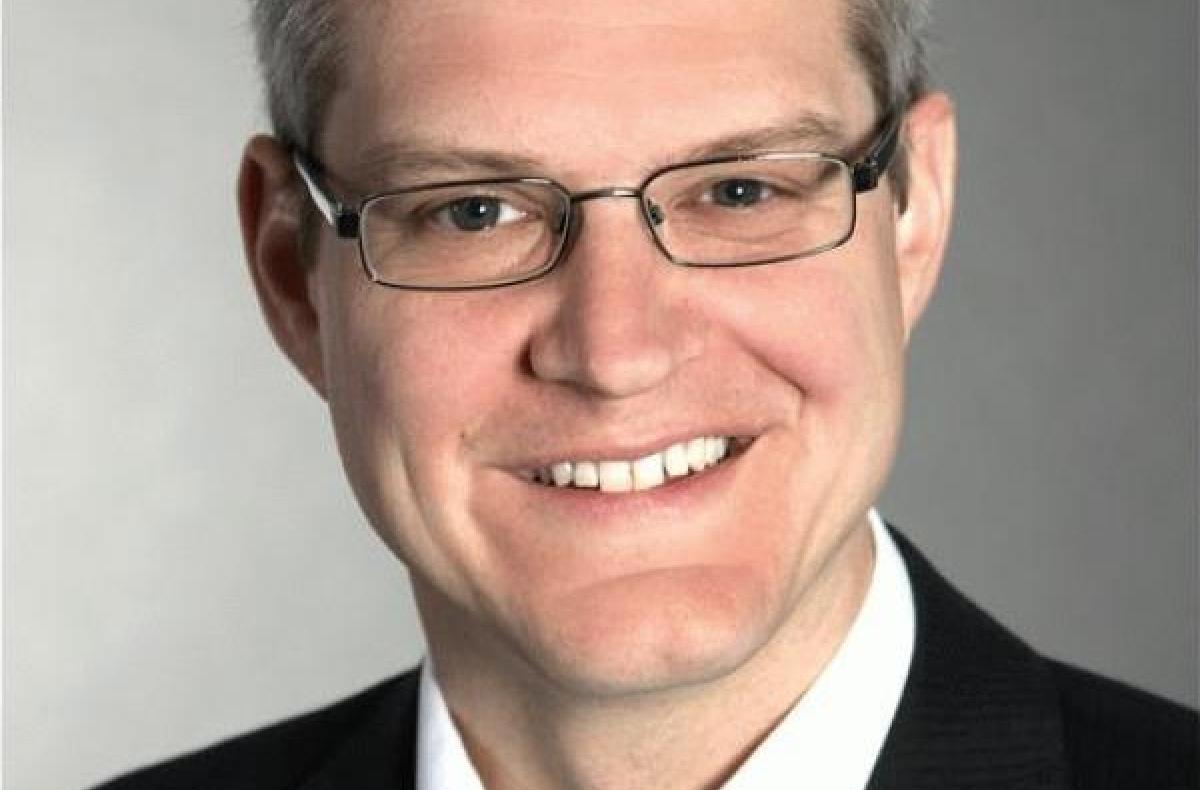
NCCR Automation: You were recently awarded the Friedrich Wilhelm Bessel Research Award by the Alexander von Humbolt Foundation. How did this come about?
Daniel Kuhn: To be honest, I didn't know the prize before. It was a colleague, Professor Steffen Rebennack from Karlsruhe, who knew about the prize nominated me. The prize is interdisciplinary so I competed with researchers from other areas, such as cancer medicine, so I initially thought my chances of winning it with my rather abstract research were quite low.
The surprise prizes are the best after all.
(laughs).
What is your research about?
Basically, I study so-called uncertain optimisation problems with my research group. So we try to optimise something under uncertain conditions.
Can you give an example?
If I want to invest capital in the stock market, I am interested in how much profit the various assets will make next year. Of course, I won't know that until next year and therefore have to decide in favour of this or that asset under uncertain conditions. In other cases, uncertainties lie in measurements. For example, if I want to optimally control the heating of a building and have to measure the room temperature with sensors. How do I deal with the fact that the measured values are always subject to a certain inaccuracy?
Sounds like a balancing act on shaky ground – a ropedance, so to speak.
So to speak. In my research, I think about all possible realisations of these uncertainties and try to optimise each situation accordingly. Often, I try to minimise the costs in the worst case.
Isn't that a somewhat pessimistic approach?
That is true. However, in my experience, even though the decision is based on the worst-case scenario, you can fair quite well with it in other cases also. In the aftermath of the 2007 financial crisis, for example, there was a lot of discussion about why so many banks went bankrupt. One of the reasons that are often given is that banks used old models to value contracts. But these models only worked under normal conditions. During a crisis situation like that, they did not deliver meaningful results. They valued the chances of bankruptcy too low. With my models, which are especially optimised for the worst case, the banks could have better protected themselves against a crisis.
Sounds like a good way to deal with a pandemic.
I myself have not applied my methods in this area, but in principle it would be possible. However, there is always a trade-off. As with an insurance policy, one pays a certain "premium" to prepare for a crisis. If the crisis does not occur, these costs were for nothing. Ultimately, it is a political question what "premium" one is willing to pay.
How does your research fit into the NCCR and how could it contribute to the "reliably automated future" that the NCCR strives for?
Within the NCCR, I am involved in the projects on regulation in a data-rich and uncertain world. These aim to use data to model uncertainty and ultimately optimise control processes. Optimised control is central to a reliably automated future.
Why?
Take security of power supply, for example. Operators of a reservoir power plant, for example, only have a limited amount of water available for electricity generation. The amount of water is influenced by the weather and therefore cannot be predicted with certainty. In addition, the demand and thus the price for the electricity varies. Now the operators have to formulate a strategy for operating the power plant - when do they produce electricity and when don't they? With our methods, we can run through all scenarios - from a full lake with continuous rain to a virtually completely evaporated puddle during a drought. This allows us to develop the best strategy for each situation and incorporate it into the control strategy. Ultimately, this results in reliable automation of power plant operation - and thus a contribution to security of power supply.