Fertile ground: How reinforcement learning can improve food sustainability

Farming is one of the most complex, unpredictable industries imaginable, with outcomes so much at the mercy of factors beyond human control. There is simply no way to control, or even reliably predict, the weather. Soil quality is affected by such a broad array of factors (from rainfall to past crops), and is so difficult and expensive to test, that it is nearly unmanageable. The main things that farmers can actively manage are crop rotation, genetics, tillage, irrigation and fertilization.
Calculating the optimal fertilisation or irrigation strategy for a particular location – given all the wildcard influences – demands calculations that are simply outside human capacity. While farmers certainly have plenty of knowledge to draw on, their calculations must be based on regional or national averages and cannot account for all the specifics of their unique field. Machines of course can handle such computation; but how can they build up sufficient data? Getting meaningful data on a specific location, with long crop cycles and ever-changing weather, could take thousands of years. Drawing from a wider pool of farms in a region shortens this timespan, but at the cost of specificity; neighbouring farms may have quite different conditions. That’s the problem NCCR Automation researcher Matteo Turchetta is addressing.
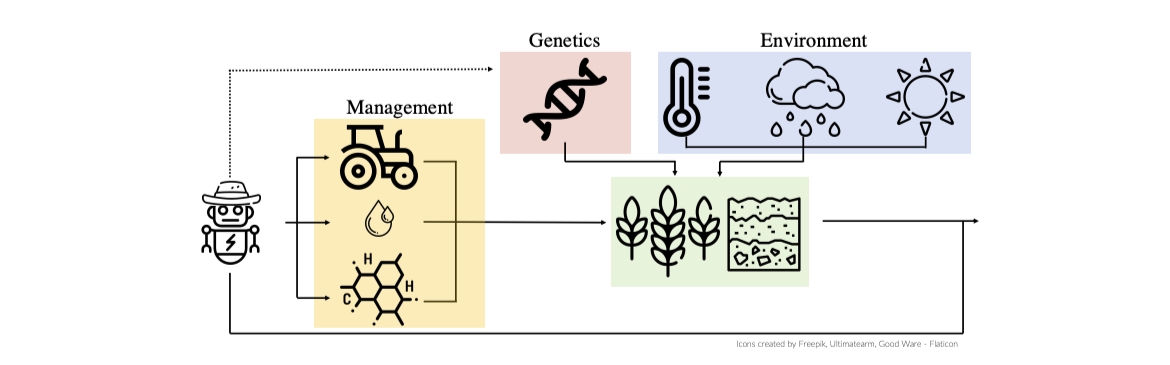
Matteo and his ETH Zurich colleague Luca Corinzia, along with Scott Sussex, who was engaged with the project at an earlier stage, have fixed their attention on fertilisation. This aspect has a double significance: first, fertilisation has a major environmental impact, in terms of water pollution and greenhouse gas emissions. And second, it can be optimised for each farm, since nitrogen in the soil is fairly mobile and, therefore, changes rapidly. So, theoretically, there is plenty of room to tailor an optimal solution for each location and reap significant benefits. In practice, determining the best practice for specific crops, climate, soil conditions and terrain is a matter of trial and error – and that simply takes too long. Unless you run those experiments through a simulation.
The digital twin solution
Reinforcement learning (RL) – the kind of machine learning that trains algorithms based on feedback from their environments – should be a productive tool to find adaptive strategies for agriculture… if only it weren’t for the long turnover times. Since effective RL demands thousands of feedback cycles, it can’t be applied to real-world agricultural data; instead, simulations can be used to test specified conditions and propose the best fertilisation strategy. But those now available restrict inputs to single dimensions: just one crop, one year, one type of crop management. Different simulators, of course, make different assumptions and simplifications, and can be useful for various purposes. But the gap between theory and practice is daunting.
Matteo, Luca and a wider team of researchers have tackled this gap with CyclesGYM: the first crop management RL environment that can accommodate multiple crops over multiple years. That really matters, because food security and sustainability are inherently long-term challenges. Heavy fertilisation might generate a rich crop this year, but degrade next year’s harvest. To nurture an ecosystem that can ensure a healthy food supply in the future as well as right now, we need to optimise crop rotation, fertilisation and other strategies over a long time period.
CyclesGYM’s back-end is based on an existing agronomic model (Cycles), but uses it to create a digital twin (fine-tuned to a specific location) that can learn adaptive fertilisation strategies. Essentially, the digital twin leverages a little data to generate a lot – even based on conditions that were not observed in reality. And with reinforcement learning, that enlarged data pool can generate actionable decisions that deliver impressive efficiency gains, reducing fertiliser waste and increasing yield.
The first real-world testing of site-specific strategies devised by CyclesGYM took place over the 2023 growing season, with the support of the Swiss centre of excellence for agricultural research, Agroscope. Four winter wheat fields in Vaud were managed according to recommendations provided by RL agents trained in CyclesGYM, and the results have generated more valuable feedback for the model. While performance in two of the fields was good (cutting costs while maintaining or slightly increasing production), the other two yielded counterintuitive results. On investigation, it turned out that one of those fields has unusually shallow soil, which seems to have affected water retention. Meanwhile, the other field enjoys proximity to a herd of cows that provide an abundance of natural fertilisation. These two examples show the kind of specific conditions that can’t be accounted for when using an off-the-peg model, but can be factored into CyclesGYM adaptation to generate more tailored strategies and better results. (In the 2024 growing season, lessons from this first experience have been incorporated into an improved system that generates more accurate models.)
Real-world benefits
Matteo and Luca are now developing an app to make it easy for farmers, advisers and researchers to get accurate, field-specific recommendations. Through KoraLabs (billed as “the AI nutritionist for your crops”), the pair have set their sights on multiple goals: they hope to improve access to smart strategy tools, especially for smaller farms; cut fertiliser costs; and at the same time, to reduce greenhouse gas emissions and environmental pollution.
Using the app, farmers will be able to easily plug in their specific conditions and current weather patterns, and get meaningful, customised strategy recommendations. No doubt adaptive planning tools like this will become ever more valuable in a changing climate, as farmers’ direct experience may no longer fit emerging conditions – that’s when data from other regions (and climates) can complement local history to provide a fuller picture. Gathering user feedback will ensure that the tool keeps up to date, keeps learning and stays relevant – enabling not only farmers, but the entire community, to harvest the benefits of lower emissions and more sustainable food.
Matteo and Luca are eager to connect with more farmers and others in the agri-food space, for exchange of ideas and experience, to gather data, and more. Anyone interested in participating in the trial, or knowing more about KoraLabs, can sign up through the website.