Carl-Johann Simon-Gabriel
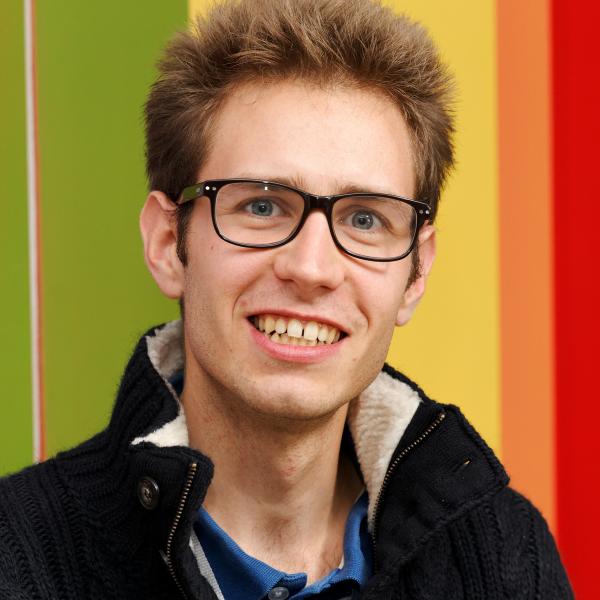
Dr.
Carl-Johann Simon-Gabriel
Alumni
NCCR Automation is a great opportunity to apply my research to energy- and environment-related domains and help the transition towards a low carbon society
Carl-Johann was a post-doc working together with Prof. Andreas Krause on adversarial images, kernel methods, reinforcement learning and causality, between August 2020 and November 2021. He is also very interested in energy and climate change related questions, and hope to be able, one day, to efficiently help reducing our carbon footprint. He did his PhD at the Max Planck Institute for Intelligent Systems in Tübingen (Germany), under the supervision of Prof. Bernhard Schölkopf, where he worked on generative image models (GAN & VAE) and their connections to well-known and/or kernel-based distribution dissimilarities (such as KL-divergences, maximum mean discrepancies, etc.).